1-10-100 Rule – The Cost of Doing Nothing
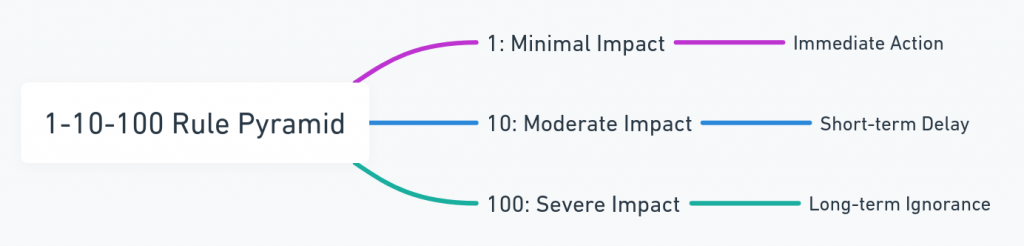
The 1-10-100 rule was developed by George Labovitz and Yu Sang Chang in 1992 and is widely used to describe efficiency. The rule is relevant to many business and personal situations as long as they involve data quality and the cost of correction. Customers and prospective customers are your business’s bedrock; ensuring you have an accurate phone number and valid email addresses to reach them is essential and will save time and money.
In this scenario, we will be looking at marketing data and how, if not managed properly, it could lead to a major source of waste and loss of profits. Consider a situation where call center staff enter high-quality contact information from live callers into a database. Did you know up to 20 percent of the contact data is flawed at entry? And if the same high-quality data is not regularly maintained by this time next year, it will have decayed by up to 40%.
1-10-100 is defined as any number of ‘units’ measured in monetary value or resource costs. In this example, the 1-10-100 rule will be expressed in dollars as applied to data quality at different stages in the data cycle, illustrating the importance of maintaining data quality regularly rather than sporadically or not at all.
The 1-10-100 rule related to data quality is:
(1) Prevention Cost – Verifying the quality or maintaining data will cost $1
It has been established it typically costs $1 to confirm contact information at the point of entry. This works as a guard and will not let the invalid data be added until the correction is made – making sure you start with clean data. This $1 includes the validation solution, as well as the expense of the hourly employee and running the computer equipment for each record. Adding a phone number verification API to your incoming data can cost less than $1.
(10) Correction Cost – If the prevention stage was skipped, correction costs occur to fix the record – this involves scrubbing and de-duping, costing the business $10
If the validation solution was applied in batch to cleanse and de-duplicate contact data after the point of entry, it was determined to cost around $10. The reason is that some data is so malformed that no intelligent validation software alone could address all the data issues. In these cases, the entries may not be able to be counted as sales leads or valid customer contacts.
(100) The Failure Cost – If nothing is done to the data and you have lost a valuable contact, your decayed record has cost $100
The cost of doing nothing at all was been shown to be $100 per record. Because of lost marketing opportunities, it simply costs more not to verify data upfront to ensure that you have valid customer connections.
The 1-10-100 rule proves that when it comes to data, earlier is preferable to later, and employing 1-10-100 allows businesses to identify problems upfront before they enter your data sphere. Once data validation at the point of entry is in place, frequent database maintenance is best to ensure no high costs are accrued due to bad data and that the data you maintain is of high quality.
The bottom line is this: It’s cheaper to identify disconnected phone numbers and execute a phone number checker at the start of your digital data collection process. Consider validating your data a required cost of doing business in the digital information age.
Article originally published:
About The Author
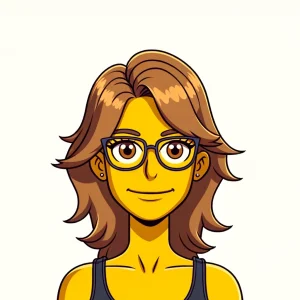